As we have already written here on our blog, the present and the future of mechanical engineering are computerized. Neural networks and other forms of Artificial Intelligence are part of our daily lives and help in several stages of our work.
Here at Vibroacustica, knowledge in machine learning and in genetic and evolutionary algorithms (genetic algorithms, evolutionary algorithm), for example, are part of the list of solutions we offer to customers, within our work with simulations to optimize time and resources.
Within artificial intelligence, neural networks are among the most advanced and can also be used in engineering. A recent example is the lifetime simulations of components, which we will briefly introduce in this text.
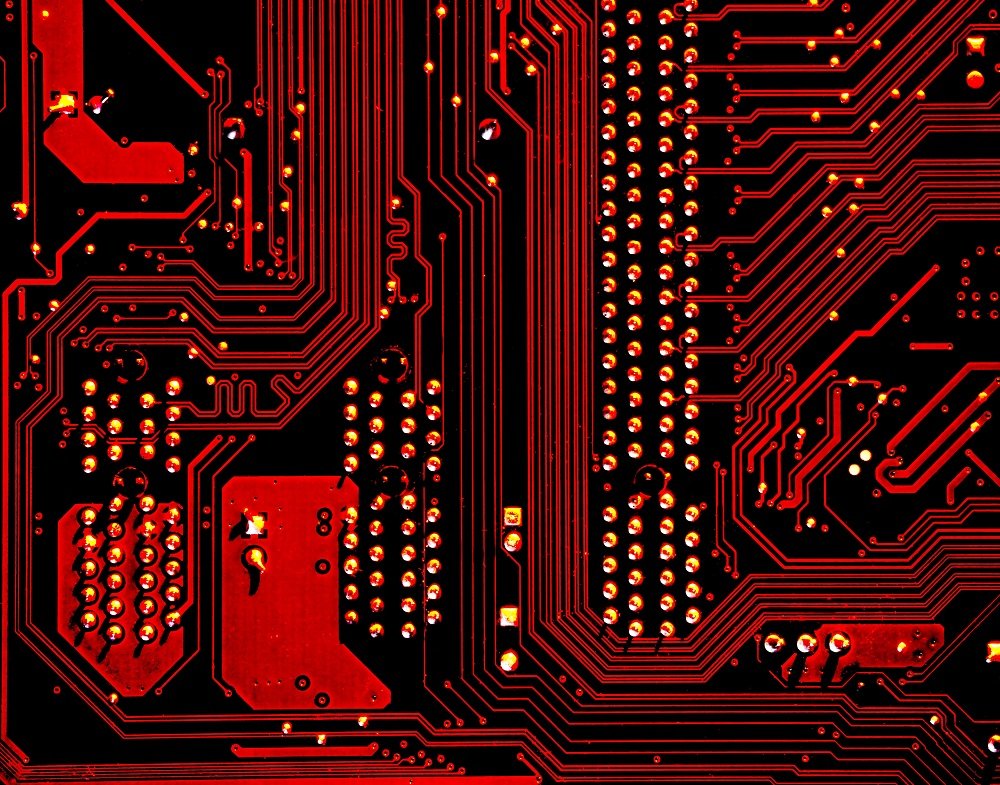
What is a neural network?
In short, neural networks are computer systems inspired by the human brain. They replicate, in machines, the way human beings learn – and just like us, they improve over time.
There are several interconnected nodes that work like neurons. Thus, they can identify patterns and find answers to complex problems with little human interference. In other words, they recognize patterns and correlations in databases.
Neural networks have been researched since the 1940s and are currently useful in various industry segments, ranging from the medical field to the chemical and, of course, engineering industries.
A recent example of the use of neural networks in our industry is a study by Osaka University in Japan, which used machine learning to predict bearing life.
Neural networks for component simulation
The developed model specifically analyzes the remaining useful life of bearings, components of various rotating equipment, such as compressors.
It combines convolutional neural networks to analyze digital images with hierarchical Bayesian regression modeling to evaluate hypotheses through statistical probability.
This system, basically, analyzes how long the equipment will work, through a relationship between damage progression x remaining useful life of the bearing.
Through the vibration spectrum of the parts, scientists can predict the remaining service life. The more the defect worsens, the greater the oscillation of the vibration amplitude.
The neural network is trained through a spectrogram with different frequencies of vibration over time, so that it is able to predict how the bearing wear will be.
The Osaka University model reduced prediction errors about remaining bearing life by 32%. In addition, by knowing more precisely when a part is going to fail, industrial machinery maintenance becomes more efficient.
You can read the full study here.
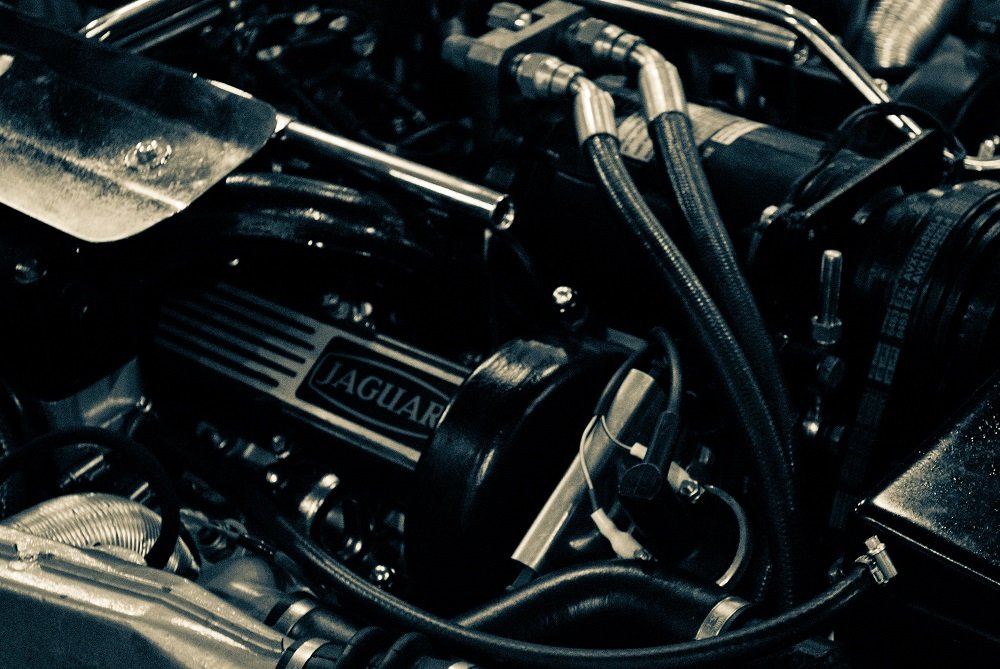
Innovation is key in the industry
Better and better solutions are developed to solve problems, whether in engineering or in other areas. Therefore, we are always up to date on the main ways to solve and predict problems in our customers’ mechanical engineering projects.
Among our efforts are important strategic partnerships, such as HBK and Randon’s CTR, one of the largest technology centers in Latin America. Find out more about our work here!
NVH Specialists
Vibroacustica is specialized in NVH analysis and simulations in several areas, in addition to having expertise in compressor projects for national and international customers.